Resonance Neural Network
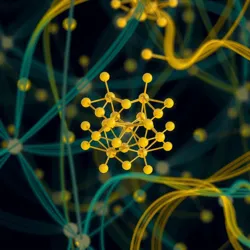
The Resonance Neural Network (RNN) is an advanced artificial intelligence algorithm developed to detect and analyze complex patterns within intricate datasets. It is particularly noted for its application in the study of mineral memory, where it has been instrumental in revealing the potential for data storage and retrieval capabilities in minerals.
Development and Background
The Resonance Neural Network was developed by a team of interdisciplinary scientists led by Dr. Elara Voss at the Geoscience Research Institute. The need for such an algorithm arose from the challenges faced in analyzing the atomic structures of minerals like quartz and calcite, which were hypothesized to have memory capabilities. These challenges required a sophisticated algorithm capable of identifying subtle and intricate patterns not easily detectable by traditional neural networks.
Functionality and Design
The RNN is designed to excel in pattern recognition tasks, utilizing a unique architecture that mimics the resonant properties observed in certain mineral structures. This architecture allows the RNN to effectively process and interpret data from complex systems, such as geological formations, by identifying patterns that indicate potential memory retention.
Key Features
- Pattern Recognition: The RNN is highly effective at detecting patterns within large and complex datasets, making it ideal for applications in geology and materials science.
- Data Processing: It can efficiently handle vast amounts of data, analyzing atomic interactions and arrangements that suggest memory capabilities.
- Integration with Other Technologies: The RNN can be integrated with existing AI frameworks, enhancing their capabilities in specialized fields such as data storage technology and environmental monitoring.
Applications
Mineral Memory
The most prominent application of the Resonance Neural Network has been in the field of mineral memory. By employing the RNN, researchers like Dr. Voss have been able to analyze mineral samples and uncover their potential for storing and retrieving information. This breakthrough has significant implications for developing new, sustainable data storage solutions.
Environmental Science
In environmental science, the RNN is used to monitor ecological changes by analyzing geological formations over extended periods. This application offers insights into long-term environmental shifts, contributing to our understanding of climate change and ecosystem dynamics.
Challenges and Future Prospects
While the Resonance Neural Network has demonstrated remarkable capabilities, it faces challenges in terms of scalability and integration into broader technological systems. Future research aims to enhance its efficiency and applicability across various scientific disciplines.
The potential for the RNN extends beyond its current applications. Researchers are exploring its use in developing self-healing materials and other innovative technologies that leverage the memory capabilities of natural materials.
See Also
- Artificial Intelligence Unveils Mineral Memory Potential
- Dr. Elara Voss: Pioneer in AI and Geology
- Revolutionary Data Storage: Minerals Take Center Stage
- Geologists and AI: A New Frontier
References
- "Resonance Neural Network: A New Approach to Pattern Recognition." AI Research Quarterly, Vol. 12, Issue 4, 2023.
- "Unlocking the Secrets of Mineral Memory with Advanced AI." Journal of Innovative Sciences, Vol. 20, Issue 6, 2023.
The Resonance Neural Network stands as a testament to the power of interdisciplinary collaboration, bridging the gap between artificial intelligence and natural sciences to unlock new possibilities in understanding and utilizing the natural world.